XLSTAT Zoom: How to become a PCA guru, with XLSTAT
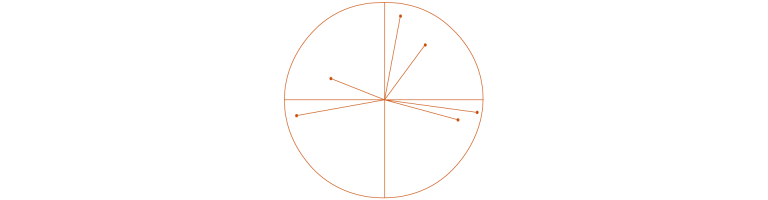
Did you know that Principal Component Analysis (PCA) is one of the most widely used XLSTAT features?
It’s not surprising as this data mining method is extensively used in marketing, biostatistics, sociology, and many other fields. With XLSTAT’s powerful algorithms, you’ll have more than 15 output tables and graphs generated automatically by XLSTAT, while more than 20 options are available to customize your PCA. But don’t worry, it’s very easy to master!
Let’s start with an easy example!
We have some data from the US Census Bureau which describe the changes in the population of 51 states between 2019 and 2020. Using PCA, we can analyze the correlations between the variables and to find out if the changes in population in some states are very different from the ones in others.
Ready for some quick theory?
Often datasets hold a lot of interesting information you want to look at. This information is likely to be spread in a homogeneous way. PCA will build an artificial dataset, with the same number of columns as the original dataset. It will then maximize the amount of information in the first few columns of the artificial data it generated.
In PCA jargon, columns created by PCA are called dimensions, or factors, or axes. Information is called inertia or variability.
For more theory, check out our video:
Among the main purposes of PCA are:
- Investigating the relationships between variables (e.g. products)
- Checking how individuals are described by variables (e.g. consumers)
- Examining proximities among individuals
- Obtain a new dataset appropriate to use in modeling methods such as linear regression
How to build a PCA in XLSTAT?
Five simple steps:
- Import your data into Excel
- Launch the PCA dialog box under Analyzing data menu
- Select your data
- Choose the options/output of your interest
- Click OK to run the PCA
An easy example is available on our Help Center.
Which PCA options can you find in XLSTAT?
- Input data: Observations/variables table, correlation matrix and covariance matrix are the three types of data format you can choose. For example, if your data correspond to a table comprising q observations described by p quantitative variables, select the Observations/variables option
- PCA type: You can choose between Correlation matrix (standardized or normalized PCA), Covariance matrix (unstandardized or non-normalized PCA) and Spearman correlation matrix.
- Standardization: If the format of your data is "observations/variables", you can choose how correlation (or covariance) will be computed: with denominator (n) or (n – 1).
- Filtering factors: Reduce the number of factors for which results are displayed by choosing either the Minimum% option (minimum percentage of the total variability that the chosen factors must represent) or Maximum number of factors option.
- Rotation: Apply a rotation (Varimax, Promax, etc) to the factor coordinate matrix.
- Add supplementary variables and observations. These observations/variables are not taken into account for the computation of the correlation matrix and for the subsequent calculations (we talk of passive observations as opposed to active observations).
- Pairwise deletion: Remove observations only when the variables involved in the calculations have missing data.
- By group analysis: Perform one PCA per group, per selected group or on merged groups.
- Hierarchical Ascending Classification: You can automatically open the pre-filled dialog box of HAC and perform a classification of the observations on the factor scores tables.
- Results: Descriptive statistics, correlation/covariance matrix, Bartlett's sphericity test, KMO measure, eigenvalues, contributions, squared cosines, factor scores,
- Graphs: Correlation chart, biplot (correlation, distance, asymmetric), observations chart, bootstrap charts.
Latest tweets
No tweet to display