Penalty analysis
Penalty analysis detects product characteristics that could be improved to increase quality. Do it in Excel using the XLSTAT add-on statistical software.
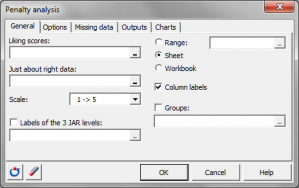
What is penalty analysis
Penalty analysis is a method used in sensory data analysis to identify potential directions for the improvement of products, on the basis of surveys performed on consumers or experts.
Two types of data are used:
- Preference data (or liking scores) that correspond to a global satisfaction index for a product (for example, liking scores on a 9 point scale for a chocolate bar), or for a characteristic of a product (for example, the comfort of a car rated from 1 to 10).
- Data collected on a JAR (Just About Right) scale. These correspond to ratings ranging from 1 to 6 for one or more characteristics of the product of interest. 1 corresponds not « Not enough at all », 2 to « Not enough », 3 to « JAR » (Just About Right), an ideal for the consumer, 4 to « Too much » and 5 to « Far too much ». For example, for a chocolate bar, one can rate the bitterness, and for the comfort of the car, the sound volume of the engine.
The method, based on multiple comparisons such as those used in ANOVA, consists in identifying, for each characteristic studied on the JAR scale, if the rankings on the JAR scale are related to significantly different results in the liking scores.
For example, if a chocolate is too bitter, does that significantly impact the liking scores?
The word penalty comes from the fact that we are looking for the characteristics which can penalize the consumer satisfaction for a given product. The penalty is the difference between the mean of the liking scores for the JAR category, and the mean of the scores for the other categories.
Principle of penalty analysis
Penalty analysis is subdivided into three phases:
- The data of the JAR scale are aggregated: on one hand, categories 1 and 2 are grouped, and on the other hand categories 4 and 5 are grouped, which leads to a three point scale. We now have three levels: "Not enough", "JAR", and "Too much".
- We then compute and compare the means of the liking scores for the three categories, to identify significant differences. The difference between the means of the 2 non-JAR categories and the JAR category is called mean drops.
- We compute the penalty and test if it is significantly different from 0.
Results for penalty analysis in XLSTAT
After the display of the basic statistics and the correlation matrix for the liking scores and the JAR data, XLSTAT displays a table that shows for each JAR dimension the frequencies for the 5 levels (or 7 or 9 depending on the selected scale). The corresponding stacked bar diagram is then displayed. The table of the collapsed data on three levels is then displayed, followed by the corresponding relative frequencies table and the stacked bar diagram. The penalty table allows you to visualize the statistics for the 3 point scale JAR data, including the means, the mean drops, the penalties and the results of the multiple comparisons tests.
Last, the summary charts enable you to quickly identify the JAR dimensions for which the differences between the JAR category and the 2 non-JAR categories ("Not enough", "Too much") are significantly different: when the difference is significant, the bars are displayed in red color, whereas they are displayed in green color when the difference is not significant. The bars are displayed in grey when the size of a group is lower than the select threshold (see the Options tab of the dialog box).
The mean drop vs % chart displays the mean drops as a function of the corresponding % of the population of testers. The threshold % of the population over which the results are considered significant is displayed with a dotted line.
analyze your data with xlstat
Included in
Related features