Propensity Score Matching
Use this feature to match participants of two distinct groups in order to control the effect of confounding variables in observational studies.
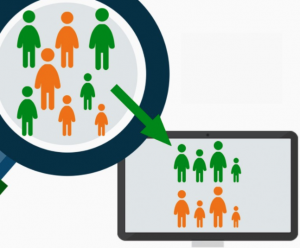
What is propensity score matching?
The propensity score is defined as the probability for a participant to belong to one of two
groups given some variables known as confounders. The propensity score matching is a
technique that attempts to reduce the possible bias associated with those confounding variables
in observational studies.
Propensity Score Matching options in XLSTAT
Once the propensity score has been estimated, each participant of the treatment group is matched to the most similar participant of the control group (Rosenbaum P. R. (1989)). The distance matrix is computed between the treatment group and the control group. XLSTAT implementation proposes two metrics: the Euclidean distance and the Mahalanobis distance.
Two algorithms are available in XLSTAT to perform the matching operation: the greedy algorithm and the optimal algorithm. With both of these algorithms, it is possible to match each participant of the treatment group to one participant of the control group, to a specified number of participants of the control group or to all participants of the control group.
Propensity Score Matching results in XLSTAT
Test of the null hypothesis: The H0 hypothesis corresponds to the independent
model which gives probability p0 whatever the values of the explanatory variables. We seek to
check if the adjusted model is significantly more powerful than this model.
Type II analysis: This table is only useful if there is more than one explanatory variable. Here,
the adjusted model is tested against a test model where the variable in the row of the table in
the question has been removed.
The table of propensity scores gives the calculated propensity score for each participant of
the two groups. The value of the logit of the propensity score is also given. This is the value
that is used to compute the distance between each participant.
The distance matrix is also displayed to give a general view of all the computed distances.
Participants of the treatment group are in rows, those of the control group are on columns.
Distances for match pairs are displayed in bold.
ROC curve: The ROC curve is used to evaluate the performance of the model by means of
the area under the curve (AUC) and to compare several models together (see the description
section for more details).
analyze your data with xlstat
Included in
Related features