Multivariate Analysis of Variance (MANOVA)
Use this model to carry out a MANOVA (Multivariate ANalysis Of VAriance) of two or more balanced or unbalanced factors.
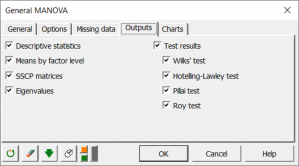
Description of the MANOVA in XLSTAT
General description
The MANOVA uses the same conceptual framework as the ANOVA. The main difference comes from the nature of the dependent variables: instead of a single one, we can study many of them. With the MANOVA, explanatory variables are often called factors. Effects of factors are estimated on a combination of several response variables.
The advantage of the MANOVA as opposed to several simultaneous ANOVAs lies in the fact that it takes into account correlations between response variables, which results in a richer use of the information contained in the data.
The MANOVA tests the presence of significant differences among combinations of levels of factors on several response variables. MANOVA also enables the simultaneous tests of all hypotheses tested by an ANOVA and is more likely to detect differences between levels of factors.
Furthermore, the computation of several ANOVAs instead of one MANOVA increases the Type I error, which is the probability that the null hypothesis will be wrongly rejected.
The potential covariation between response variables is not taken into account with several ANOVAs. Instead, the MANOVA is sensitive to both the difference of averages between levels of factors and the covariation between explanatory variables. And a potential correlation between response variables is more likely to be detected when these variables are studied together, as is the case with a MANOVA.
Interactions
An “interaction” is an artificial factor (not measured) that reflects the interaction between at least two measured factors. For example, if we carry out treatment on a plant, and tests are carried out under two different light intensities, we will be able to include in the model an interaction factor treatment*light which will be used to identify a possible interaction between the two factors. If there is an interaction between the two factors, we will observe a significantly larger effect on the plants when the light is strong and the treatment is of type 2, while the effect is average for weak light, treatment 2 and strong light, treatment 1 combinations.
To make a parallel with linear regression, the interactions are equivalent to the products between the continuous explanatory values, although here obtaining interactions requires nothing more than simple multiplication between two variables. However, the notation used to represent the interaction between factor A and factor B is A*B.
The interactions to be used in the model can be easily defined in XLSTAT.
Balanced and unbalanced MANOVA
We speak about balanced MANOVA when the numbers of categories are equal for all combinations of factors. When the numbers of all categories for one of the combinations of factors are not equal, then the MANOVA is said to be unbalanced. XLSTAT can handle both cases.
Constraints
During the calculations, each factor is broken down into a sub-matrix containing as many columns as there are categories in the factor. Typically, this is a full disjunctive table. Nevertheless, the breakdown poses a problem: if there are gg categories, the rank of this sub-matrix is not g but g−1. This leads to the mandatory deletion of one of the columns of the sub-matrix and possibly the transformation the other columns. The strategy taken in XLSTAT is the following:
a1=0: the parameter for the first category is null. This choice allows us to force the effect of the first category as a standard. In this case, the constant of the model is equal to the mean of the dependent variable for group 1.
Moreover, the number of observations should be equal to at least the sum of the number of dependent variables and the number of factors and interactions included in the model (+1).
Multivariate tests
One of the main application of the MANOVA is multivariate comparison testing where parameters for the various categories of a factor are tested to be significantly different or not. For example, in the case where four treatments are applied to plants, we want to know if treatments have a significant effect and also if treatments have different effects.
Numerous tests have been proposed to compare means of each category. Most of them rely on the relationships that exist between the error matrix E and the matrix symbolizing the tested hypotheses H that is the eigenvalues of the matrix E−1. XLSTAT provides the main tests including:
- Wilks Lambda test
- Hotelling-Lawley’s Trace test
- Pillai’s Trace test
- Roy’s greatest root test
The last 3 tests detailed above (Hotelling-Lawley’s Trace test, Pillai’s Trace test, Roy’s greatest root test) are less used than the Wilks’ Lambda test and are based on the Fisher distribution for calculating p-values.
Results of the MANOVA in XLSTAT
Summary statistics: The tables of descriptive statistics show the simple statistics for all the variables selected. The number of observations, missing values, the number of non-missing values, the mean and the standard deviation (unbiased) are displayed for the dependent variables (in blue) and the quantitative explanatory variables. For qualitative explanatory variables, the names of the various categories are displayed together with their respective frequencies.
Means of level factor: This table provides for each level factor and quantitative variable the mean value.
SSCP matrices: These tables are displayed to give a general view of the effects of the factors and interactions between factors.
Wilks’ test (Rao’s approximation): This table provides the results of Wilks' Lambda test which tests the hypothesis of equality of the mean vectors for the different levels.
Hotelling-Lawley test: This table provides the results of Hotelling-Lawley trace test, which tests the hypothesis of equality of the mean vectors for the different levels.
Pillai’s test: This table provides the results of Pillai’s trace test, which tests the hypothesis of equality of the mean vectors for the the different levels.
Roy’s test: This table provides the results of Roy’s greatest root test which tests the hypothesis of equality of the mean vectors for the different levels.
Example
A tutorial on one-way MANOVA is available on the Addinsoft website.
analyze your data with xlstat
Related features